-
Notifications
You must be signed in to change notification settings - Fork 1k
Commit
This commit does not belong to any branch on this repository, and may belong to a fork outside of the repository.
- Loading branch information
Showing
126 changed files
with
1,321 additions
and
395 deletions.
There are no files selected for viewing
This file was deleted.
Oops, something went wrong.
This file contains bidirectional Unicode text that may be interpreted or compiled differently than what appears below. To review, open the file in an editor that reveals hidden Unicode characters.
Learn more about bidirectional Unicode characters
This file contains bidirectional Unicode text that may be interpreted or compiled differently than what appears below. To review, open the file in an editor that reveals hidden Unicode characters.
Learn more about bidirectional Unicode characters
This file contains bidirectional Unicode text that may be interpreted or compiled differently than what appears below. To review, open the file in an editor that reveals hidden Unicode characters.
Learn more about bidirectional Unicode characters
Loading
Sorry, something went wrong. Reload?
Sorry, we cannot display this file.
Sorry, this file is invalid so it cannot be displayed.
Loading
Sorry, something went wrong. Reload?
Sorry, we cannot display this file.
Sorry, this file is invalid so it cannot be displayed.
This file contains bidirectional Unicode text that may be interpreted or compiled differently than what appears below. To review, open the file in an editor that reveals hidden Unicode characters.
Learn more about bidirectional Unicode characters
Binary file not shown.
This file contains bidirectional Unicode text that may be interpreted or compiled differently than what appears below. To review, open the file in an editor that reveals hidden Unicode characters.
Learn more about bidirectional Unicode characters
Original file line number | Diff line number | Diff line change |
---|---|---|
@@ -0,0 +1,48 @@ | ||
{ | ||
"cells": [ | ||
{ | ||
"cell_type": "code", | ||
"execution_count": null, | ||
"metadata": {}, | ||
"outputs": [], | ||
"source": [ | ||
"!pip install vanna\n", | ||
"import vanna\n", | ||
"from vanna.remote import VannaDefault\n", | ||
"vn = VannaDefault(model='chinook', api_key=vanna.get_api_key('[email protected]'))\n", | ||
"vn.connect_to_sqlite('https://vanna.ai/Chinook.sqlite')\n", | ||
"vn.ask(\"What are the top 10 albums by sales?\")" | ||
] | ||
}, | ||
{ | ||
"cell_type": "code", | ||
"execution_count": null, | ||
"metadata": {}, | ||
"outputs": [], | ||
"source": [ | ||
"from vanna.flask import VannaFlaskApp\n", | ||
"VannaFlaskApp(vn).run()" | ||
] | ||
}, | ||
{ | ||
"cell_type": "markdown", | ||
"metadata": {}, | ||
"source": [ | ||
"## Here's what you'll get\n", | ||
"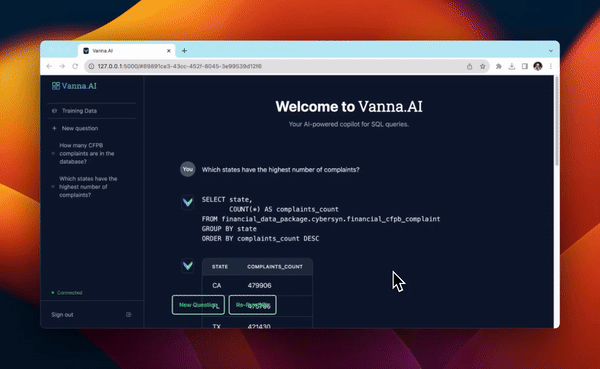" | ||
] | ||
}, | ||
{ | ||
"cell_type": "markdown", | ||
"metadata": {}, | ||
"source": [] | ||
} | ||
], | ||
"metadata": { | ||
"language_info": { | ||
"name": "python" | ||
} | ||
}, | ||
"nbformat": 4, | ||
"nbformat_minor": 2 | ||
} |
Oops, something went wrong.