We read every piece of feedback, and take your input very seriously.
To see all available qualifiers, see our documentation.
Have a question about this project? Sign up for a free GitHub account to open an issue and contact its maintainers and the community.
By clicking “Sign up for GitHub”, you agree to our terms of service and privacy statement. We’ll occasionally send you account related emails.
Already on GitHub? Sign in to your account
Hello @mdancho84, Here is reproducible codes for the error. I am using modeltime.h2o with modeltime resamples
library(Quandl)
library(modeltime.ensemble) library(modeltime) library(tidymodels)
library(glmnet) library(xgboost)
library(tidyverse) library(lubridate) library(timetk)
library(modeltime.h2o) library(tidymodels) library(h2o) h2o.init() h2o.removeAll() df1 <- Quandl(code = "FRED/PINCOME", type = "raw", collapse = "monthly", order = "asc", end_date="2017-12-31") df2 <- Quandl(code = "FRED/GDP", type = "raw", collapse = "monthly", order = "asc", end_date="2017-12-31")
per <- df1 %>% rename(PI = Value)%>% select(-Date) gdp <- df2 %>% rename(GDP = Value)
data <- cbind(gdp,per) data1 <- tk_augment_differences( .data = data, .value = GDP:PI, .lags = 1, .differences = 1, .log = TRUE, .names = "auto") %>% select(-GDP,-PI) %>%
rename(GDP = GDP_lag1_diff1,PI = PI_lag1_diff1) %>% drop_na()
horizon <- 6 lag_period <- 6 rolling_periods <- c(10:12) data_pre_full <- data1 %>%
#bind_rows(
#) %>%
tk_augment_lags( .value = GDP : PI , .lags = lag_period)
%>%
tk_augment_slidify( .value = PI_lag6, .period = rolling_periods, .f = mean, .align = "center", .partial = TRUE)
data_prepared_tbl <- data_pre_full %>%
filter(!is.na(GDP)) %>% dplyr::select(-PI) %>% drop_na()
splits <- time_series_split(data_prepared_tbl, assess = 8, cumulative = TRUE)
recipe_spec <- recipe(GDP~ ., data = training(splits)) # %>%
train_tbl <- rsample::training(splits) %>% bake(prep(recipe_spec), .) test_tbl <- rsample::testing(splits) %>% bake(prep(recipe_spec), .)
model_spec <- automl_reg(mode = 'regression') %>% parsnip::set_engine( engine = 'h2o', max_runtime_secs = 99999999999999999, max_runtime_secs_per_model = 3600, project_name = 'project_01', nfolds = 0, max_models = 2, #exclude_algos = c("DeepLearning"), include_algos = c("GLM"), seed = 786 )
model_fitted <- model_spec %>%
fit(GDP ~ ., data = training(splits))
leaderboard <- automl_leaderboard(model_fitted) leaderboard
model2 <- leaderboard$model_id[[1]] model_fit_2 <- automl_update_model(model_fitted, model2)
calibration_tbl <- modeltime_table( model_fit_2)
resample_spec <- rolling_origin( data_prepared_tbl, initial = 100, assess = 6, cumulative = TRUE, skip = 0, lag = 0, overlap = 0 )
resamples_fitted <- calibration_tbl %>% modeltime_fit_resamples( resamples = resample_spec , control = control_resamples(verbose = TRUE))
resamples_fitted %>% modeltime_resample_accuracy( metric_set = metric_set(rmse, rsq))
The text was updated successfully, but these errors were encountered:
No branches or pull requests
Hello @mdancho84, Here is reproducible codes for the error. I am using modeltime.h2o with modeltime resamples
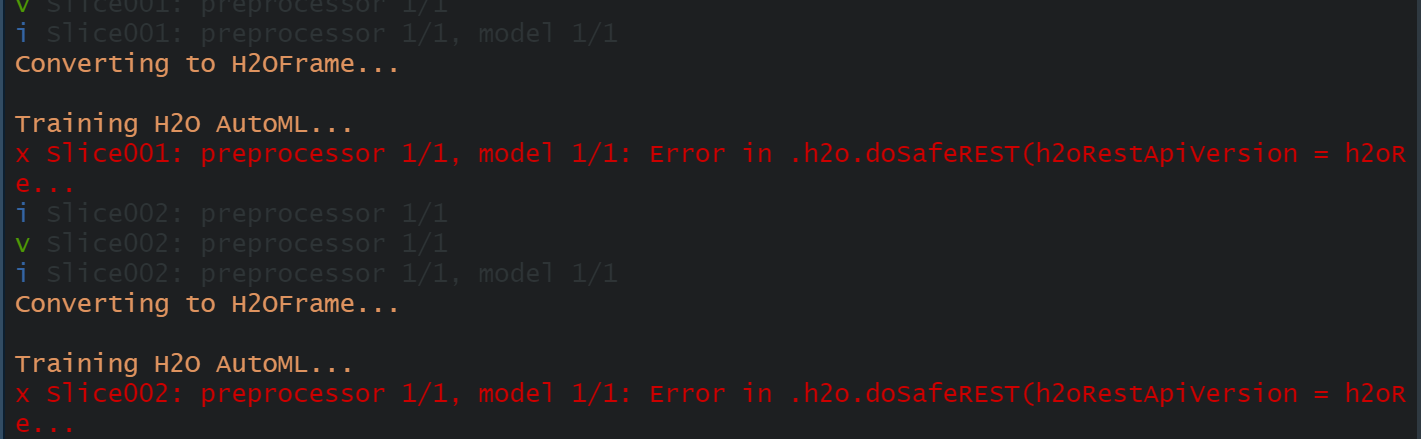
library(Quandl)
Tidymodeling
library(modeltime.ensemble)
library(modeltime)
library(tidymodels)
Base Models
library(glmnet)
library(xgboost)
Core Packages
library(tidyverse)
library(lubridate)
library(timetk)
library(modeltime.h2o)
library(tidymodels)
library(h2o)
h2o.init()
h2o.removeAll()
df1 <- Quandl(code = "FRED/PINCOME",
type = "raw",
collapse = "monthly",
order = "asc",
end_date="2017-12-31")
df2 <- Quandl(code = "FRED/GDP",
type = "raw",
collapse = "monthly",
order = "asc",
end_date="2017-12-31")
per <- df1 %>% rename(PI = Value)%>% select(-Date)
gdp <- df2 %>% rename(GDP = Value)
data <- cbind(gdp,per)
data1 <- tk_augment_differences(
.data = data,
.value = GDP:PI,
.lags = 1,
.differences = 1,
.log = TRUE,
.names = "auto") %>%
select(-GDP,-PI) %>%
rename(GDP = GDP_lag1_diff1,PI = PI_lag1_diff1) %>%
drop_na()
horizon <- 6
lag_period <- 6
rolling_periods <- c(10:12)
data_pre_full <- data1 %>%
Add future window----
#bind_rows(
future_frame(.data = .,.date_var = Date, .length_out = horizon)
#) %>%
add lags----
tk_augment_lags(
.value = GDP : PI ,
.lags = lag_period)
%>%
add lag rolling averages
tk_augment_slidify(
.value = PI_lag6,
.period = rolling_periods,
.f = mean,
.align = "center",
.partial = TRUE)
2.0 STEP 2 - SEPARATE INTO MODELING & FORECAST DATA ----
data_prepared_tbl <- data_pre_full %>%
filter(!is.na(GDP)) %>%
dplyr::select(-PI) %>%
drop_na()
splits <- time_series_split(data_prepared_tbl, assess = 8, cumulative = TRUE)
recipe_spec <- recipe(GDP~ ., data = training(splits)) # %>%
train_tbl <- rsample::training(splits) %>% bake(prep(recipe_spec), .)
test_tbl <- rsample::testing(splits) %>% bake(prep(recipe_spec), .)
MODEL SPEC ----
model_spec <- automl_reg(mode = 'regression') %>%
parsnip::set_engine(
engine = 'h2o',
max_runtime_secs = 99999999999999999,
max_runtime_secs_per_model = 3600,
project_name = 'project_01',
nfolds = 0,
max_models = 2,
#exclude_algos = c("DeepLearning"),
include_algos = c("GLM"),
seed = 786
)
model_fitted <- model_spec %>%
fit(GDP ~ ., data = training(splits))
leaderboard <- automl_leaderboard(model_fitted)
leaderboard
model2 <- leaderboard$model_id[[1]]
model_fit_2 <- automl_update_model(model_fitted, model2)
MODELTIME ----
calibration_tbl <- modeltime_table(
model_fit_2)
resample_spec <- rolling_origin(
data_prepared_tbl,
initial = 100,
assess = 6,
cumulative = TRUE,
skip = 0,
lag = 0,
overlap = 0
)
resamples_fitted <- calibration_tbl %>%
modeltime_fit_resamples(
resamples = resample_spec ,
control = control_resamples(verbose = TRUE))
resamples_fitted %>%
modeltime_resample_accuracy(
metric_set = metric_set(rmse, rsq))
The text was updated successfully, but these errors were encountered: