-
Notifications
You must be signed in to change notification settings - Fork 2
/
Copy path02-data-handling.Rmd
359 lines (246 loc) · 11.5 KB
/
02-data-handling.Rmd
1
2
3
4
5
6
7
8
9
10
11
12
13
14
15
16
17
18
19
20
21
22
23
24
25
26
27
28
29
30
31
32
33
34
35
36
37
38
39
40
41
42
43
44
45
46
47
48
49
50
51
52
53
54
55
56
57
58
59
60
61
62
63
64
65
66
67
68
69
70
71
72
73
74
75
76
77
78
79
80
81
82
83
84
85
86
87
88
89
90
91
92
93
94
95
96
97
98
99
100
101
102
103
104
105
106
107
108
109
110
111
112
113
114
115
116
117
118
119
120
121
122
123
124
125
126
127
128
129
130
131
132
133
134
135
136
137
138
139
140
141
142
143
144
145
146
147
148
149
150
151
152
153
154
155
156
157
158
159
160
161
162
163
164
165
166
167
168
169
170
171
172
173
174
175
176
177
178
179
180
181
182
183
184
185
186
187
188
189
190
191
192
193
194
195
196
197
198
199
200
201
202
203
204
205
206
207
208
209
210
211
212
213
214
215
216
217
218
219
220
221
222
223
224
225
226
227
228
229
230
231
232
233
234
235
236
237
238
239
240
241
242
243
244
245
246
247
248
249
250
251
252
253
254
255
256
257
258
259
260
261
262
263
264
265
266
267
268
269
270
271
272
273
274
275
276
277
278
279
280
281
282
283
284
285
286
287
288
289
290
291
292
293
294
295
296
297
298
299
300
301
302
303
304
305
306
307
308
309
310
311
312
313
314
315
316
317
318
319
320
321
322
323
324
325
326
327
328
329
330
331
332
333
334
335
336
337
338
339
340
341
342
343
344
345
346
347
348
349
350
351
352
353
354
355
356
357
358
359
# Data handling with R
## Data types
- numeric:
- `2`
- `12.125`
- character strings: `'a'`, `"word"`
- logical:
- `TRUE`
- `FALSE`
- `NULL` (sort of)
:::: {.infobox .note data-latex="note"}
Please note that logicals are in capital letters.
::::
- vectors:
- `c(12, 15, 35698)`
- `c("FOSS4G 2022", "Firenze")`
- list: `list(1, 45, 12.0, "toto")`
- matrices: `matrix(0:9, 3,3)`
- data frames (df): `data.frame(x = 1:3, y = c("a", "b", "c"))`
- constants:
- `letters[3:4]`
- `LETTERS[12:26]`
- `pi`
## Assignment
<!--jn: what do you mean by "affectation"?
It is French for assignment ;)-->
Use of variables to store data in memory.
Use `<-` (or `=`)
Examples:
```{r eval=FALSE}
a <- c(0, 1, 2) # new integer vector
b <- c("FOSS4G", "2022", "Firenze") # new character vector
c <- data.frame(
number = a,
strings = b)
d <- list(1, 45, 12.0, "toto") # lists can store different data types
e = matrix(0:9, 3,3)
```
## Not only a calculator
R is shipped with lots of functions like :
```{r r-functions, eval=FALSE}
data(<datasetname>) # load an embedded dataset
head(<objectname>) # first lines of a dataframe
is.vector(object) # return TRUE if object is a vector
is.data.frame(object) # return TRUE if object is a data.frame
class(<objectname>) # returns the class of an object
unique(<objectname>) # returns unique values
help(<functionname>) # get help on a function
plot(<objectname>) # create a graphic from a dataset
```
Exercise:
- Load the `LakeHuron` dataset using `data()`
- Get help on the `LakeHuron` dataset
- Use `head()` to see its first lines
- Plot `LakeHuron`
## Packages
While base R contains a lot of functions, it can be extended with various packages.
> In R, the fundamental unit of shareable code is the package. A package bundles together code, data, documentation, and tests, and is easy to share with others. @wickham2022Packages
You already saw how to install packages with the `install.packages()` function, let's see how to load the [{dplyr}](https://dplyr.tidyverse.org/)
package we will use to handle the data.
```{r data-handling-libraries}
#| message: false
library(dplyr)
```
## Load data
If the packages do not provide datasets to work with, you will want to work on your own data.
There is several ways to load data.
Base R provides a set of functions for delimited text files.
For example, if we want to work with the Gapminder dataset from [Software Carpentry's R course](https://github.com/swcarpentry/r-novice-gapminder).
```{r data-handling-download-gapminder}
data_url <- "https://raw.githubusercontent.com/swcarpentry/r-novice-gapminder/main/data/gapminder_data.csv"
# download the data and store it in a variable
gapminder <- read.csv(data_url)
```
We call this dataset from an URL but it can be a path in your system file.
For example, if you have installed the {gapminder} package as recommended, you will find the data set as a TSV file in your R installation.
```{r where-is-gapminder}
data_url <- system.file("extdata/gapminder.tsv", package = "gapminder")
data_url
```
:::: {.infobox .tip data-latex="tip"}
`system.file()` is a function that returns the path of files in R packages, independently
of the operating system.
::::
```{r load-gapminder-from-package, eval=FALSE}
gapminder <- read.delim(data_url, sep = "\t")
```
:::: {.infobox .tip data-latex="tip"}
`read.csv()` and similar functions can read delimited text files only.
For other formats, you can use functions from other packages like [{haven}](https://haven.tidyverse.org) or [{readxl}](https://readxl.tidyverse.org/).
We will show in Chapter \@ref(rspatial) how to load geospatial data.
::::
Let's take a look to our data :
```{r data-handling-glimpse}
glimpse(gapminder)
```
This dataset is about life expectancy, population and GDP per capita in world countries between `r min(gapminder$year)` and `r max(gapminder$year)`.
You can also use `head()` for the same purpose but a different output format.
```{r data-handling-head}
head(gapminder)
```
We can transform a data frame into a tibble to access their better `print()` method that combines `head()` and `glimpse()`.
Tibbles are at the core of the Tidyverse packages.
```{r data-handling-head2}
gapminder <- as_tibble(gapminder)
gapminder
```
:::: {.infobox .note data-latex="note"}
If you want more information about the `gapminder` dataset, Hans Rosling made a [TED talk](https://www.ted.com/talks/hans_rosling_the_best_stats_you_ve_ever_seen) presenting the gapminder data.
::::
We can see that it contains several columns (also called *variables*):
```{r data-handling-names}
names(gapminder)
```
## In the beginning was the Verb
{dplyr} provides a lot of functions to handle data: `filter()` to filter the data matching certain conditions (subset data), `select()` to select columns, `mutate()` to create new variables.
All those functions are verbs and means an action onto the dataset.
## Filter data
If we only want the records from Italy, we can filter using:
```{r data-handling-filter}
filter(gapminder, country == "Italy" )
```
:::: {.infobox .tip data-latex="tip"}
in Tidyverse functions, the data is the first argument and does not need to be named.
::::
You can use comparison operators like `==`, `>`, `<`, `>=` , etc.
There is also logical operators : `&` (*AND*), `|` (*OR*), `!` (*NOT*) and `xor()`.
So, for example, if we want to subset records for Italy after 2000, we can use filter like this:
```{r data-handling-subset-italy}
italy_2000 <- filter(gapminder, country == "Italy" & year > 2000 )
italy_2000
```
This can be translated to : "From the gapminder dataset, filter all rows where the country is equal to Italy and the year is superior to 2000".
### Exercise {-}
- Try to subset non-European records
- Try to subset records that are in Oceania or before 2000
## Select columns
`select()` allows you to keep only the columns you need for your analysis.
Maybe we only want the `country`, `year` and `lifeExp` variables:
```{r data-handling-select}
select(italy_2000, country, year, lifeExp)
```
Or, for example, in the `italy_2000` subset, the `continent` variable does not provide useful information anymore, so we want to discard it.
Please not the use of the `-` symbol before the name of the variable.
```{r data-handling-select2}
select(italy_2000, -continent)
```
So you can select the column you want to keep or the ones you want to remove.
## Create new variables
Let say you want to compute the GDP, in millions, from the population and the GDP per capita variables.
For that, you can use the `mutate()` function:
```{r data-handling-mutate}
mutate(gapminder, GDP = (gdpPercap * pop) / 1000000)
```
It's companion function, `transmute()` does the same thing but only keep the new variables.
```{r data-handling-transmute}
transmute(gapminder, GDP = (gdpPercap * pop) / 1000000)
```
If you want to keep variables from the dataset, you can call them in the function call:
```{r data-handling-transmute2}
transmute(gapminder, country, year, GDP = (gdpPercap * pop)/ 1000000)
```
So in this example, we compute the GDP but we also keep the information about the country and the year.
## Agregate data
Sometimes, we have a too detailed dataset and we want a more broader view of the data so we want to aggregate it.
To do so, {dplyr} provides a couple of functions: `group_by()` and `summarise()`.
Like their names say, `group_by()` groups records which share the same value in a variable and `summarise()` compute the summary of non-grouping variables.
For example, let's compute the yearly population of each continent.
To do this, we first group the data by continent and year and pass the result to `summarise()`.
In this second function, we create a new variable called `population` that is the sum of the variable `pop` of each group.
```{r data-handling-group-by}
summarise(group_by(gapminder, continent, year), population = sum(pop))
```
You can use any function to summarise your data, for example, if you want to know the number of entries by continent, you can use `n()`.
```{r data-handling-summarise}
summarise(group_by(gapminder, continent), count = n())
```
Or if you only want the first country of each continent:
```{r data-handling-summarise2}
summarise(group_by(gapminder, continent), country = first(.data[["country"]]))
```
:::: {.infobox .note data-latex="note"}
`.data` is a pronoun that you can use when the column name is a character vector.
::::
### Exercise {-}
- Determine the max GDP by country other the period (tip: there is a `max()` function base R.)
## Join data
{dplyr} provides a large variety of functions to join datasets :
`inner_join()`,`left_join()`, `right_join()`, `full_join()`, `nest_join()` ,
`semi_join()`,`anti_join()`.
Let's create two datasets that shares a common key.
This new key will be the country name and the year separated by an underscore.
```{r data-handling-left}
gapminder_left <- transmute(gapminder,
key = paste0(country, "_" , year),
country,
continent,
year)
gapminder_left
```
```{r data-handling-right}
gapminder_right <- transmute(gapminder,
key = paste0(country, "_" , year),
lifeExp,
pop,
gdpPercap)
gapminder_right
```
Now we can join them.
```{r}
joined_gapminder <- left_join(
gapminder_left,
gapminder_right,
by = "key" # optional argument if the join variables have the same name
)
```
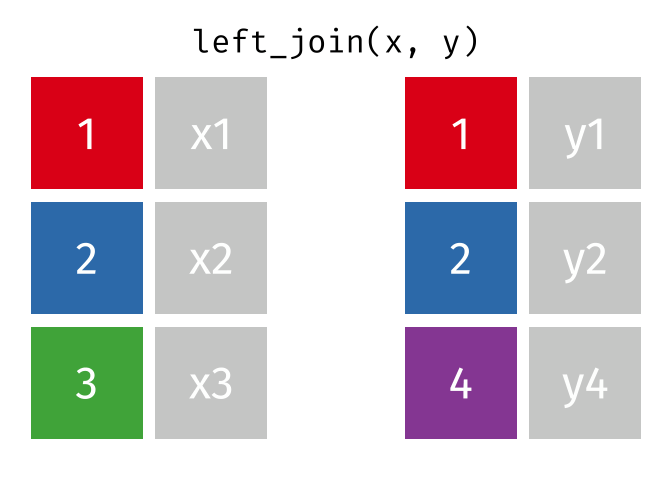
If you want more information on joins operations with {dplyr}, we recommend to read the [dedicated blogpost from Garrick Aden-Buie](https://www.garrickadenbuie.com/project/tidyexplain/)
## Piping
Piping allows to create a sequence of actions on a dataset without storing intermediate results.
As it can be difficult to debug piped commands for beginners, we won't use it in this workshop.
However, its usage is very frequent so it is most likely that a beginner will encounter it in documentation or in code source publicly available.
The most common form `%>%` is provided by the package [{magrittr}](https://magrittr.tidyverse.org/), which is part of the tidyverse and is a dependency of {dplyr}, so you don't have to load it.
In the following example, we show how to compute the mean GDP by the decade of European countries using pipes to chain functions.
```{r piping-example-magrittr}
gapminder %>% # pass the dataset as the first argument
filter(continent == "Europe") %>% # subset on European records
select(-continent) %>% # remove the continent column
mutate(decade = year - year %% 10, # compute decade
GDP = (gdpPercap * pop) / 1000000 # compute GDP
) %>%
group_by(country, decade) %>% # grouping variables
summarise(mean_GDP = mean(GDP)) # compute mean GDP of the decade
```
With R 4.1.0, the built-in piping operator `|>` has been introduced:
```{r piping-example-Rbase}
gapminder |> # pass the datset as the first argument
filter(continent == "Europe") |> # subset on European records
select(-continent) |> # remove the continent column
mutate(decade = year - year %% 10, # compute decade
GDP = (gdpPercap * pop) / 1000000 # compute GDP
) |>
group_by(country, decade) |> # grouping variables
summarise(mean_GDP = mean(GDP)) # compute mean GDP of the decade
```
Those two pipes operators are not equivalent so we recommend to read this [R-bloggers' blogpost](https://www.r-bloggers.com/2021/05/the-new-r-pipe/) on pipe's operators.